Explainability and transparency
There are lots of benefits to value explainability and transparency, especially as a company. .
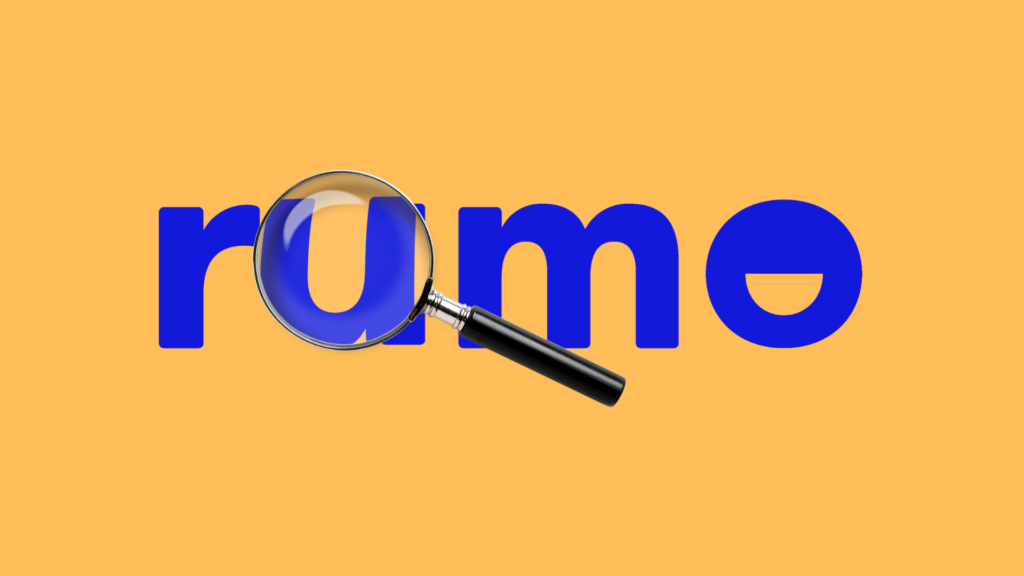
Explainability and transparency are Rumo’s core values. Not only does it answer lots of modern ethical questions, but it also has many advantages. By transparency, we mean giving end users the keys to understand the recommendation process. Our main objective is to make clear the ins and outs rather than exhaustively explaining each feature and details of our algorithm.
Why explainabiltiy ?
By allowing end-users to understand the recommendation process, we allow them to take ownership of it. Therefore, they use it even better. In “Too much, too little, or just right? Ways explanations impact end users’ mental models” authors Todd Kulesza, Simone Stumpf, Margaret Burnett, Sherry Yang, Irwin Kwan and Weng-Keen Wong come to the conclusion that the surprise-explain-reward method is the most effective.
You may be wondering how this works? Well, it leverages curiosity by surprising users, then explains the benefits of the behavior it is trying to encourage. It’s the user’s reward for investing their attention.
The goal is to convince end users that spending a little time discovering a new feature is a worthwhile investment. In the case of recommender systems, it is useful to be able to control the system to get even more accurate recommendations. However, not all users need the same features explained to them: it is necessary to identify what is worth highlighting on an individual level. Users must be able to sense the benefits of the proposed functionality, otherwise they won’t pay attention to it.
The most crucial step in the explicability process is to find the right balance between “soundness” and “completeness”. Soundness is defined in the paper as “Nothing but truth. The extent to which each component of the content of an explanation is truthful in describing the underlying system” and completeness as “All truth. The extent to which the entire underlying system is described by the explanation.”
A weak soundness might be too far off and not representative of reality, while a weak completeness might not be accurate enough and even be misleading. The conclusion of their experiment is that a high level of soundness and completeness is the best solution. The authors were surprised by this result because they did not expect that end users would prefer the longer and more complex explanation.
Gaining customer trust is vital
Users are often skeptical of recommender systems, which they see as a corporate tool to force people to consume. You can’t blame them, because these tools are indeed developed to reduce churn and increase profits. But that’s not all: they also help end-users to efficiently find what they need or want. They also broaden their perspectives and provide a much better user experience.
Ultimately, end users should be able to trust a recommendation system completely, so they would be more likely to trust the recommendation and watch, buy, read, listen, etc.
This is where transparency comes in handy. Being transparent means proving to end users that the algorithm does not take advantage of their personal data. Rumo’s recommendations are only based on interactions on the website. They are stored and compiled to build a “semantic profile” of the user and used as the basis for their recommendation.
By enabling end users to understand how content is recommended to them, they themselves become part of it and help improve it. They are more aware of the parameters involved and navigate websites more easily. As a result, they will be more likely to like content, add it to their playlists and interact with content in general. It’s a virtuous circle.
Explainability increases trust. Trust makes end users undoubting about a recommendation’s purpose. Trustful and trusted recommendations, the key to success.
Sources:
“Too Much, Too Little, or Just Right? Ways Explanations Impact End Users’ Mental Models”